无论外界怎样,请平心静气地努力!
文章目录
- 前言
- 1. 概述
- 2. 版本
- 2.1 山东青岛,2021年3月29日,Version1
- 一、climte indices库中的indices.spei()的输入和输出
- 二、基于climate_indices库计算站点SPEI
- 1. 数据准备
- 2. 站点特定尺度的spei的计算
- 3. 和spei.exe计算结果的比较
前言
此系列博文的目的是基于Python的Climate Indices库计算标准化降水蒸散发(SPEI)指数。
1. 概述
此篇博文的目的是基于Climate Indices库中计算SPEI的函数,在一个站点上展开某一时间尺度的SPEI的计算。
2. 版本
2.1 山东青岛,2021年3月29日,Version1
一、climte indices库中的indices.spei()的输入和输出
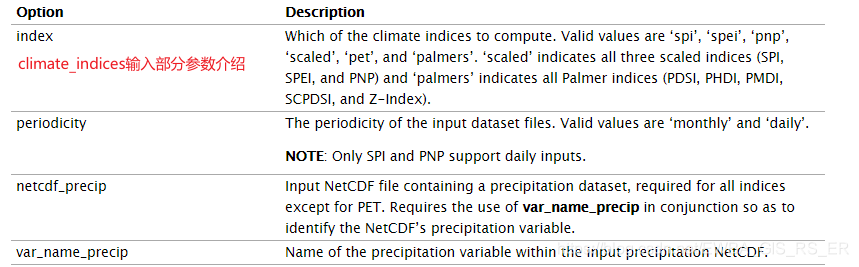
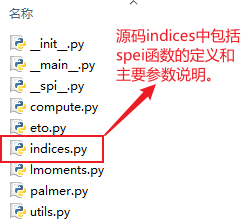
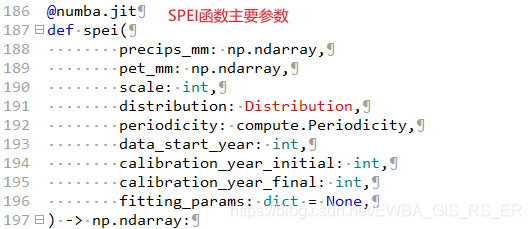
- 输入参数简要说明
参数 | 含义 | 输入格式 |
---|---|---|
precips_mm | 降水(毫米) | numpy数组 |
pet_mm | 潜在蒸散发(毫米) | numpy数组 |
scale | 时间尺度 | 整型 |
distribution | 用于拟合时间序列的分布函数 | 字符串 |
periodicity | 计算的时间单元 | 字符串,‘monthly’或’daily’ |
data_start_year | 计算开始年 | 整型 |
calibration_year_initial | 校准期最初年份 | 整型 |
calibration_year_final | 校准期最后年份 | 整型 |
param fitting_params | 预先计算的分布拟合参数的可选字典 | 字典,通常使用默认值 |
- 输出数据 计算完成之后,函数的输出数据是就是特定时间尺度的SPEI,以浮点型的np.array的格式输出。
二、基于climate_indices库计算站点SPEI
1. 数据准备
计算spei的测试数据,我们选择SPEI提出者给出的测试数据tampa.txt,选择次数的另一个好处是我们可以将基于climate indices库计算的spei和基于官方的spei.exe计算的spei进行对比。需要注意的是tampa.txt的存储格式是按照spei.exe计算时需要的格式存储的,所以下载完成之后,可以先将其进行预处理,转存到.csv文件中。
2. 站点特定尺度的spei的计算
在了解climate indices库中spei函数的基本用法之后,我们可以利用该库计算spei,由于我们利用的数据的内容是温度和降水,这里我们需要先依据桑斯维特方法计算潜在蒸散发(pet),可以再climate indices库中调用计算pet的函数直接计算pet。整个基于climate indices库计算特定时间尺度的spei的程序如下:
# -*- coding: utf-8 -*-
"""
1. 程序目的
(1) 基于climate indices库计算spei
2. 版本
2.1 山东青岛 2021年3月29日 Version 1
"""
# 相关包的导入
import numpy as np
import pandas as pd
from climate_indices import indices
from climate_indices import compute # 计算SPEI的包
# 路径处理和基本变量定义
rootdir = r'D:\SPEI_Cal\\';
tampa_file = rootdir + '01_Data\\tampa.csv'
outpath = rootdir + '03_Result\\'
lat = 27.96
styr = 1900
edyr = 2007
# 气象数据读取
tampa_data = pd.read_csv(tampa_file)
pre_data = np.asarray(tampa_data['Pre']) # 降水数据转换为np.array
tas_data = np.asarray(tampa_data['Tas']) # 温度数据转换为np.array
# 潜在蒸散发计算-桑斯维特方法
pet_data = indices.pet(temperature_celsius=tas_data,
latitude_degrees=lat,
data_start_year=styr)
# 计算SPEI
spei = indices.spei(precips_mm=pre_data,
pet_mm=tas_data,
scale=3, # 3个月尺度
distribution=indices.Distribution.gamma,
periodicity=compute.Periodicity.monthly,
data_start_year=styr,
calibration_year_initial=styr,
calibration_year_final=edyr,
)
spei[np.isnan(spei)] = -99 # nan转换为-99
spei_df = pd.DataFrame(data=spei,columns=['SPEI_1']) # 计算结果转换为DataFrame
# 计算结果写出
spei_df.to_csv(outpath+'SPEI3.csv',index=False)
print('Finished.')
3. 和spei.exe计算结果的比较
通过比较基于spei.exe和基于climate indices库计算的spei可以看出两者存在着一定的差别,这可能是由于分布拟合参数上有一些区别导致的。